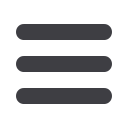
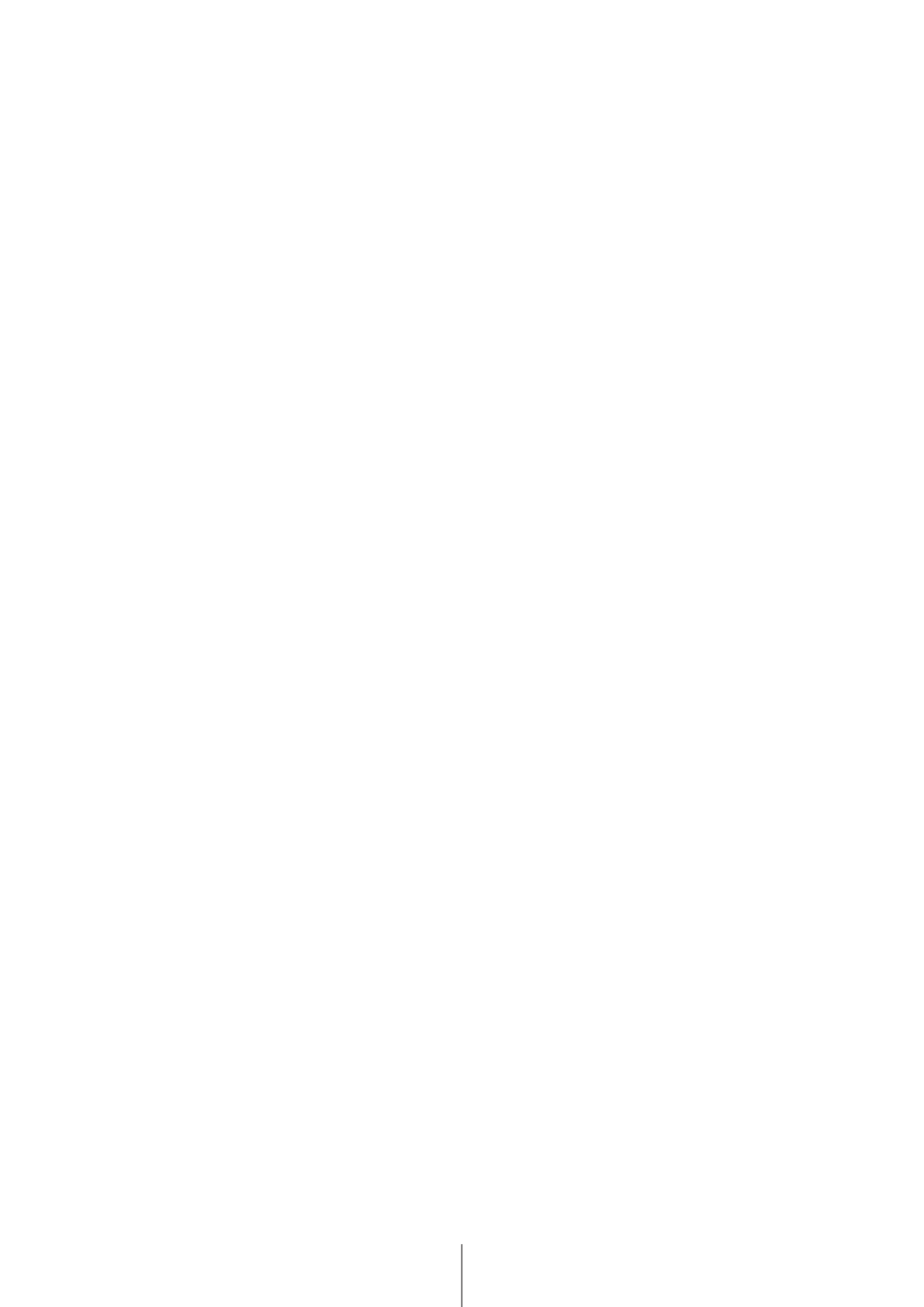
38
Artigo Original
tion applied at the nursing ward level, in order to raise
awareness, at real-time, regarding hand hygiene com-
pliance, individual behavior change and performance
optimization.The gamification solution aims at collect-
ing data from nurses procedures, provides real-time ac-
curate feedback to the nurses (the information is shown
in a screen at the nursing office). Involving nurses in
the design process of aligning the combined automated
monitoring systems with gamification with the nursing
processes, allowed a better understanding of their needs
and of the barriers facing nursing work at the ward [8].
ePharmaCare is a decision-supporting system to collab-
oratively manage medicine prescription and patient in-
formations in community pharmacies linked with online
patients.The systems supports an online pharmaceutical
service, implemented to bring the pharmacist closer to
the patient for medicine follow-up services (e.g. quick
identification of adverse events or the need for a new
prescription), valued by both [9]. Pharmacists’ lack of
time, improper time management and incurrect infor-
mation systems usage skills were the main barriers for
the full system adoption.Training, communication with
online patients and pharmacy services reorganization are
critical to ensure the correct implementation of the ser-
vice [10].
The use of decision-making algorithms is essential to
enable quick a proper use of the information for de-
cision-making. In these cases, the algorithms were de-
signed in close collaboration between researchers and
healthcare professionals and leveraging evidence-based
rules. The use of machine-learning is another level of
smart-decision-making, which will enable the delivery
of predictive models that can provide important infor-
mation to help urgent decision-making. Using machine-
learning generated epidemic patterns, the system will
be able to combine different data and issue an early epi-
demics alert. The system’s algorithms could integrate
national and international guidelines, norms and laws,
as another layer of possible analysis and guided action.
The system could provide valuable forecast capabilities
and use cognitive computing to detect patterns associ-
ated with malaria epidemics (e.g. by using IBM-Watson
Discovery Advisor functionalities) providing the right
context to smooth quick decision-making.This capabil-
ity needs to be comprehensively tested and its accuracy
properly measured (and corrected if necessary). The
systems requires as much as possible previous epidem-
ics situations in order to learn and be prepared to new
cases.
Information visualization models are also very impor-
tant.When new technology innovations are combined,
and assigning new features to a system, it allows a refin-
ing of the information, as well as a better performance
is expected.Timely reporting malaria cases is key, but it
is currently very fragile. It often depends on the profes-
sional’s availability and motivation. Hence, healthcare
people may benefit from improved working processes
and extra motivation to improve the case reporting.
The use of gamification (e.g., adapting tasks into the
form of a game to engage professional into improved
performance) could be particularly useful to improve
data entry quality since it depends on the full attention
the healthcare professional. Basic gamification func-
tionalities can be applied collaboratively with users to
encourage both ownership and behavior change, im-
proved resilience and awareness of the time-lapse from
information reception to action. It even can be linked
to a more transparent quality supported incentive
program in a ltter stage of the implementation. In the
same line, a "nudging technique" performance could
help guiding the professionals throughout the preven-
tion and control procedures, like in the OSYRISH pro-
ject, to promote nurses’ hand hygiene compliance self-
awareness and action.
The system’s approach to implementation should
strengthen the availability of information, its integra-
tion process as well as the decision-makers capacity to
act accordingly.The collaboration process is fundamen-
tal to establish a responsibility model so that they can
address regular quality processes improvement.The HP
should lead the construction of the system as they will
be using it, in real-time, for decision-making, assuring
it offers both safety and accurate procedures [11]. The
collaborative design process enables the alignment with
malaria elimination decision-making processes. It also
should allow to validade the confidence in making deci-
sions and if the system is an effective anchor to reduce
malaria at the end of the day.
Machine-learning systems are still not mature but im-
proving. The success of such a system depends on the
number of previous cases and on how elimination ac-
tions will be improved in real settings (i.e. if the systems
output are truly valuable to improve decision-making).
The organizational aspects are critical, since the system
only works if people are trainned and ready to use it.
Therefore, co-designed science research methodology
is a valuable to both engage health professionals and use
evidence-based knowledge in the design of innovative
systems that are designed to properly respond to what
specific public health professionals need. Additionally,
using a Lean approach, involving both management and
health practitioners, it will provide the understanding
wheather if the working processes are effectively de-
livering as promised, therefore benefiting the organiza-
tion by eliminating waste directly related to informa-
tion access, treatment and analysis, for example, reduc-
ing information errors or improving on quality of data
presentation [12].